Uncategorized Sunday, 2025/04/13
A team at Brigham and Women’s Hospital in the USA developed a new “clock” that may help uncover what contributes to aging. They’ve introduced a novel form of epigenetic clock, a machine learning model created to predict biological age from DNA structure. This new model differentiates between genes that retard aging and those accelerating it, accurately predicting biological age and assessing the efficacy of anti-aging interventions. Their findings were published online in Nature Aging, in a paper titled “Causality-enriched epigenetic age uncouples damage and adaptation”.
Dr. Vadim Gladyshev, the study’s senior author, emphasized that previous clocks solely considered the relationship between methylation patterns and known aging markers, failing to identify factors that cause varying aging rates. Their new clock can distinguish between changes accelerating aging and those mitigating it, predicting biological age while evaluating anti-aging interventions.
Researchers have long understood the link between DNA methylation and its impact on aging. Specific areas in DNA, known as CpG sites, have been notably correlated with aging. Lifestyle choices, such as smoking and diet, affect DNA methylation, explaining why individuals with similar lifestyles may age at different rates.
Existing epigenetic clocks can predict biological age using DNA methylation patterns. However, they cannot currently separate the methylation differences responsible for biological aging from those merely associated with the aging process.
Kejun (Albert) Ying, a graduate student, utilized a large genetic dataset to perform an epigenome-wide Mendelian Randomization (EWMR) analysis on 20,509 CpG sites with causal links to eight aging-related traits. EWMR is a technique for randomizing data and establishing causal relationships between DNA structures and observable traits.
Our Related Products
Cat.No. # | Product Name | Source (Host) | Species | Tag | Protein Length | Price |
---|---|---|---|---|---|---|
Kit-0299 | DNA Methylation Kit | Non |
![]() |
|||
Kit-0455 | Histone H3-K27 Methylation Assay Kit | Non |
![]() |
|||
Kit-0456 | Histone H3-K27 Tri-Methylation Assay Kit | Non |
![]() |
|||
Kit-0457 | Histone H3-K4 Methylation Assay Kit | Non |
![]() |
|||
Kit-0458 | Histone H3-K9 Methylation Assay Kit | Non |
![]() |
These aging-related traits include lifespan, extreme longevity (defined as survival above the 90th percentile), healthspan (the age of onset for major age-related diseases), frailty index (assessing frailty through the accumulation of health deficiencies over a lifetime), self-rated health, and three broad aging-related measures encompassing family history, socioeconomic status, and additional health factors.
Using these traits and related DNA sites, Ying developed three models: CausAge (a universal clock predicting biological age based on DNA causal factors), DamAge, and AdaptAge (including only damaging or protective changes). The authors analyzed blood samples from 7,036 individuals aged 18 to 93 in the “Generation Scotland Cohort,” ultimately training their models using data from 2,664 individuals in this cohort.
This data enabled the authors to map human CpG sites directly influencing biological aging. Scientists can use this map to identify biomarkers of aging and evaluate how different interventions contribute to longevity or accelerate aging.
The authors validated their clocks using data from 4,651 individuals in the Framingham Heart Study and Normative Aging Study. They found DamAge associated with adverse outcomes, like mortality, while AdaptAge linked to longevity, indicating age-related damage influences mortality risk, but protective DNA methylation changes may promote longevity.
They further tested their clocks’ ability to assess biological age by reprogramming stem cells (transforming specialized cells like skin cells back into a youthful, less defined state, allowing them to develop into various cell types). Applying their clocks to these newly transformed cells, DamAge decreased, indicating reduced age-related damage during reprogramming, while AdaptAge showed no specific pattern.
Finally, the authors tested their clocks’ performance on biological samples from patients with chronic conditions like cancer and hypertension and samples damaged by lifestyle factors such as smoking. In cases of age-related damage, DamAge consistently increased, whereas AdaptAge decreased, effectively capturing protective adaptations.
Gladyshev stated, “Aging is a complex process, and we still don’t quite understand the impact of anti-aging interventions. Our findings advance aging research, allowing us to more accurately quantify biological age and evaluate the lifespan-extending potential of novel anti-aging interventions.”
Classic epigenetic clock model
Horvath Clock
Currently, the Horvath epigenetic clock is the most widely used epigenetic clock, supported by extensive data in various fields such as cancer, Alzheimer’s disease, aging, and lifestyle. It is the first multi-tissue age estimator that utilizes DNA from multiple cells, tissues, and organs across different life stages. Based on the Illumina 27k or Illumina 450k arrays, methylation data was obtained from 7,844 non-cancerous samples from children and adults to assess DNA methylation levels across 51 different tissues and cell types, thereby constructing the epigenetic clock for multiple tissues and cell types. This clock was created based on 353 age-related CpGs automatically selected by mathematical algorithms, with 193 CpGs positively correlated with age and another 160 CpGs negatively correlated with age. The penalized regression model uses a logarithmic transformation version of ages 0 to 20 as the outcome measure, to better fit linear data. Its correlation between estimated age and chronological age is 0.96. The model is highly accurate, long-lived, and applicable to various tissues and cell types (covering almost all organs, including whole blood, cerebellum, colon, kidney, liver, lungs, etc.).
Hannum Clock
The Hannum clock is an age estimator based on single-tissue DNA methylation, constructed from 450K methylation data of whole blood samples from 482 Caucasian and 174 Hispanic adults. It is based on 71 age-related CpGs and uses elastic net regression to predict individuals’ chronological age. Its correlation between estimated age and chronological age is 0.96.
Since this model was trained only on adult whole blood samples, there is a significant bias estimate when applied to non-blood tissues and children’s samples. Compared to Horvath’s epigenetic clock, the Hannum epigenetic clock shows higher accuracy in predicting adult blood samples.
DNAm PhenoAge Clock
The first generation of epigenetic clocks showed weak correlation with clinical characteristics such as blood pressure, lipids, and glucose. To integrate these clinical biomarkers, an epigenetic clock called “DNA PhenoAge” was developed, using clinical data from the third U.S. National Health and Nutrition Examination Survey (NHANES III) combined with 10 clinical features, including chronological age, albumin, creatinine, glucose, C-reactive protein levels, lymphocyte percentage, mean cell volume, red cell distribution width, alkaline phosphatase, and white blood cell count. A penalized regression model was then used to regress these data on blood DNA methylation levels. Based on 513 age-related CpGs across 3 chips (27K, 450K, 850K), cross-platform applicability was achieved. DNAm PhenoAge can differentiate morbidity and mortality risk among individuals of the same chronological age. Compared to the Horvath clock, it shows significant advantages in predicting 10-year and 20-year mortality, with even stronger associations with behavioral and lifestyle factors. DNA PhenoAge also shows strong correlation with gene expression, such as increased activation of pro-inflammatory and interferon pathways, reduced activation of transcription and translation mechanisms, DNA damage response, and nuclear-mitochondrial characteristics.
DNAm GrimAge Clock
The GrimAge clock consists of 7 DNA methylation-based plasma protein markers and smoking pack-years, which have been shown to associate with morbidity or mortality. Data from 2,356 individuals’ blood samples from the Framingham Heart Study (FHS) offspring cohort were utilized, with an elastic net Cox regression model regressing the biomarkers to time of death. These biomarkers reflect inflammation, cardiovascular disease, kidney function, and cognitive function. GrimAge focuses more on lifestyle and age-related diseases, thus better predicting lifespan compared to other DNAm-based age estimators. In a twin follow-up study, GrimAge was demonstrated to be a strong predictor of mortality independent of genetic influences.
Comparison
The number of age-related CpGs varies among different epigenetic clocks (Horvath: 353, Hannum: 71, DNAm PhenoAge: 513, DNAm GrimAge: 1030), and the selection of CpGs is also different. Among these clocks, the Horvath and Hannum clocks were trained only on chronological age, while the PhenoAge and GrimAge clocks were specifically trained on composite biomarkers to predict biological age and mortality risk, therefore the latter two are referred to as chronological age-trained clocks or mortality clocks.
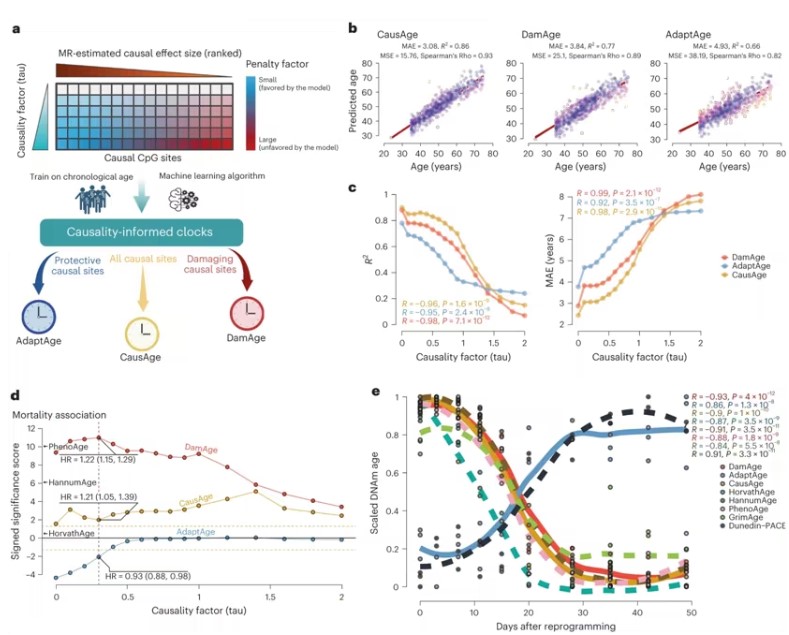
Horvath Clock
The multi-tissue DNAm age estimator (blue line in the diagram below) stands out in terms of its correlation with actual age across various tissue types, high accuracy in children, and strong correlation with gestational age in neuron cell culture models.
DNAm PhenoAge Clock
The DNAm-Phenotype (green line in the diagram below) stands out in its accuracy in predicting time to death, correlation with smoking status, and correlation with various immunosenescence markers.
Hannum Clock
The single-tissue age clock (red line in the diagram below) outperforms other blood-based biomarkers in longevity prediction.
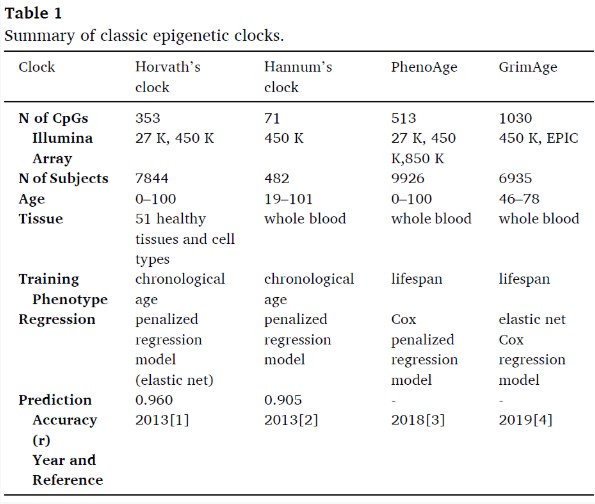
Conclusion
Measurement results of accelerated epigenetic age in blood are associated with increased natural mortality risk. If epigenetic age is greater than chronological age, it is called epigenetic age acceleration; if epigenetic age is less than chronological age, it is called epigenetic age deceleration. The deviation between chronological age and epigenetic age is one of the hotspots in the field of aging research.
Epigenetic clocks have ushered in a new era of molecular research in the field of aging, but we know little about how epigenetic clocks mediate the relationship between phenotypic manifestations of aging and chronological age. To address this issue, further longitudinal cohort studies are needed.
Existing epigenetic clocks differ not only in their CpGs spectra but also in tissue origins and racial diversity, leading to significant heterogeneity between studies and limiting applications. Therefore, future epidemiological studies may consider adopting easily accessible DNA sources for more reliable and large-scale biological age estimation.
Another limitation of epigenetic research is the difference between single cells, whether at the individual or tissue level. Sporadic senescent cells in aging tissues have been shown to be potential drivers of organ function decline. To this end, new insights into the aging process and age-related pathologies will require a shift from the homogeneity of large cell source data to the heterogeneity of single-cell analysis. In the epigenome atlas, to what extent the discretization of cell types and clock-like behavior is cell-autonomous.
Related Products & Services
- DNA methyltransferases (DNMTs)
- Epigenetics
- Biomarker
- Epigenetic Kits
- Protein Methylation Assay
- Methylation Interference Assay
Reference
- Kejun Ying et al. Causality-enriched epigenetic age uncouples damage and adaptation. Nature Aging, 2024, doi:10.1038/s43587-023-00557-0.
- New epigenetic clocks reinvent how we measure age. https://medicalxpress.com/news/2024-02-epigenetic-clocks-reinvent-age.html
- Wu Z, Zhang W, Qu J, et al. Emerging epigenetic insights into aging mechanisms and interventions[J]. Trends Pharmacol Sci. 2024;45(2):157-172.
- Duan R, Fu Q, Sun Y, et al. Epigenetic clock: A promising biomarker and practical tool in aging[J]. Ageing Res Rev. 2022;81:101743.
- Liu Z, Leung D, Thrush K, et al. Underlying features of epigenetic aging clocks in vivo and in vitro[J]. Aging Cell. 2020;19(10):e13229.
- Horvath S, Raj K. DNA methylation-based biomarkers and the epigenetic clock theory of ageing[J]. Nat Rev Genet. 2018;19(6):371-384.